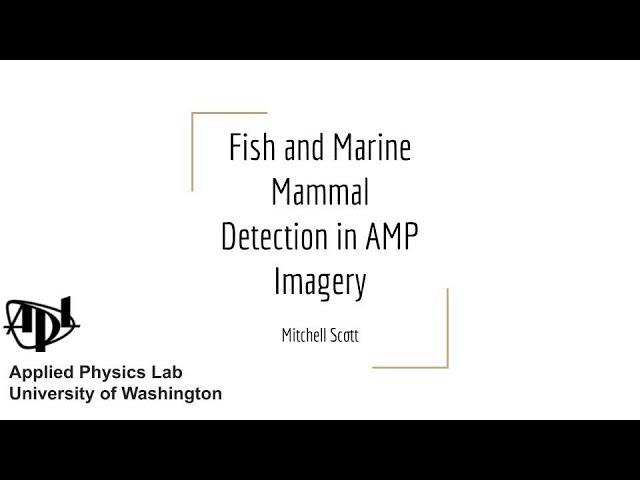
In this talk, Mitchell will present an approach to the detection and segmentation of marine animals from AMP observations that utilizes the the popular You Only Look Once (YOLO) instance segmentation algorithm, as well as traditional computer vision processing approaches. He will compare the performance of models built from data collected during AMP deployments in Sequim, WA and the Wave Energy Test Site (WETS) in Kaneohe Bay, HI, and from additional data collected at traditional hydroelectric dams. Preliminary results suggest that the current approach is adept at detecting fish while maintaining a low false positive rate.
Speaker Bio: Mitchell Scott is a software engineer at the University of Washington Applied Physics Laboratory (APL). His primary focuses include computer vision for underwater perception and marine robotic mapping, localization, and reconstruction. Prior to joining APL in 2018, he attended Washington State University where he received his B.S. (2016) and M.S. (2018) degrees in mechanical engineering, where his graduate study focused on multi-robot systems.
For more videos from the AMP, visit
For more information about PMEC, visit
0 Comments